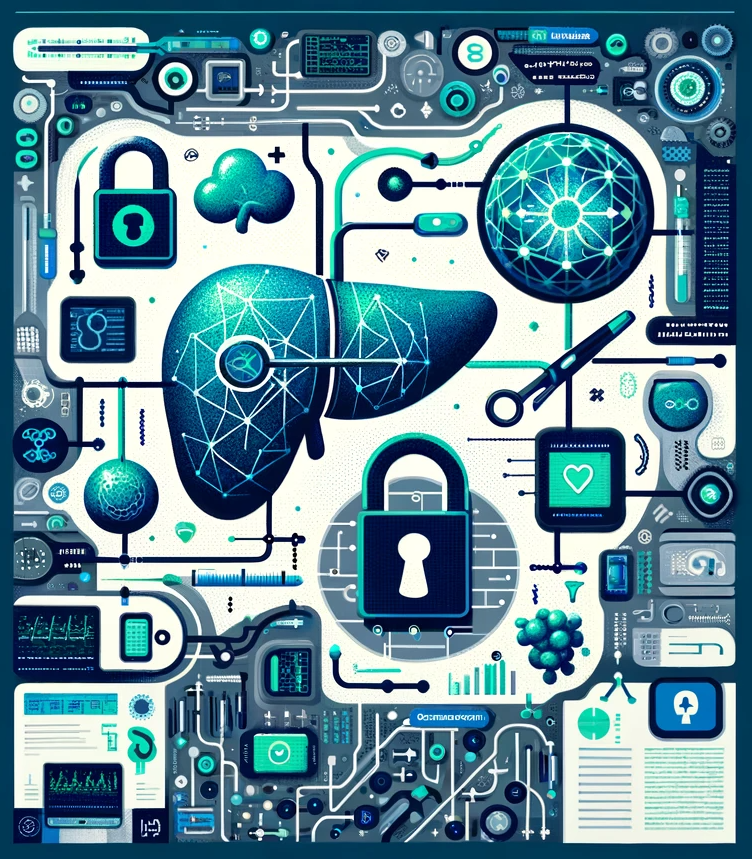
Artificial intelligence for better therapies. The research team now wants to analyze this data together. The software system that medical IT specialists develop on this basis should be able to estimate the probability of success for specific treatment approaches. Since the data comes from three different locations, the calculations go beyond the boundaries of one location. In the future, the system will be usable regardless of location. In addition, it aims to identify important parameters that influence the response to a specific treatment. By using the data collection from all three locations, the researchers want to find general factors for the success of therapy against pancreatic cancer. They see their work as an important step towards precision medicine supported by artificial intelligence.
In the long term, patients with pancreatic cancer, but also oncologists, should benefit from the treasures of data. The analyzed data can help make treatment decisions and thus potentially increase the chances of survival for patients with pancreatic cancer. In addition, those involved in the “FAIrPaCT” research network want to achieve a better understanding of the development of tumors in the pancreas and thus also the molecular mechanisms that lead to the success or failure of a treatment. On the one hand, they can create the conditions for the development of new personalized therapies. On the other hand, they want to prove that the use of software systems based on federated AI can significantly support both science and clinical practice. The federated learning methods developed by “FAIrPaCT” should also be able to be used for other oncological diseases in the future.
“FAIrPaCT” is one of eight research associations that are conducting research in the Decade Against Cancer as part of BMBF funding for interdisciplinary projects to develop and test new approaches to data analysis and data sharing in cancer research . The aim of this funding measure is to give more scientists in the field of data analysis low-threshold access to existing data from cancer research and routine oncological care. The use of newly developed approaches to data analysis should help ensure that research-relevant information can be filtered and utilized even better from existing data sets. At the same time, the culture of data sharing for research purposes should be promoted. The researched use cases can also serve as best practice solutions for data sharing in cancer research.