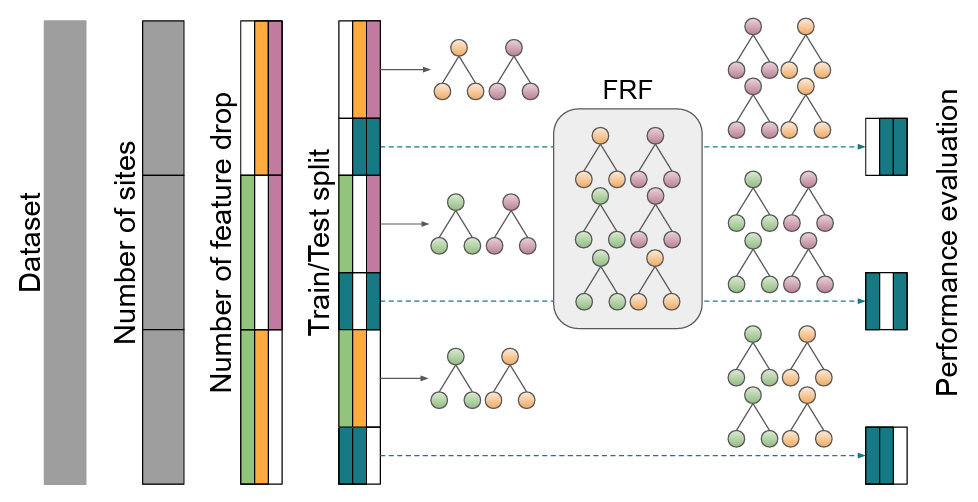
Authors: Youngjun Park, Cord Eric Schmidt, Benedikt Marcel Batton, Anne-Christin Hauschild (FAIrPaCT Member)
Introduction At FAIrPaCT, we are committed to advancing ethical AI solutions that protect data privacy while fostering scientific collaboration. Our members have contributed to a groundbreaking study on Federated Random Forest (FRF) for Partially Overlapping Clinical Data, an approach that enhances machine learning models for healthcare without compromising sensitive patient information.
The Challenge of Clinical Data Sharing In the medical field, data privacy regulations like the General Data Protection Regulation (GDPR) make it challenging to share clinical data across institutions. Moreover, healthcare datasets are often heterogeneous, meaning that features (such as test results or patient history) may not fully overlap across different hospitals. Traditional machine learning models struggle with these gaps, requiring a novel approach to address them.
What is Federated Learning? Federated Learning (FL) enables multiple institutions to train models collaboratively without sharing raw data. Instead of centralizing patient records, each institution trains a model locally and only exchanges model parameters, preserving privacy while still benefiting from a larger dataset.
Advancing FL with Federated Random Forest (FRF) This study extends Random Forest (RF) models to a federated setting where data features partially overlap between institutions. The proposed Federated Random Forest (FRF) methodology allows hospitals to:
- Train local decision trees using their available data.
- Share model updates without exposing sensitive patient records.
- Build a global model that benefits from collective knowledge across sites.
- Overcome data imbalance and missing features.
Key Findings The research was tested on three real-world clinical datasets:
- Indian Liver Patient Dataset (ILPD) – Predicting liver disease.
- Hepatocellular Carcinoma (HCC) – Identifying liver cancer.
- Breast Cancer Diagnosis (BCD) – Classifying breast tumors.
Results demonstrated that:
Federated models consistently outperformed local models, even with missing features. More participating hospitals led to better predictions, while local models suffered from data scarcity.
The additive aggregation method improved results, enabling more robust collaboration. Even with partial feature overlap, FRF provided significant gains in predictive accuracy.
Why This Matters for FAIrPaCT This research directly aligns with FAIrPaCT’s mission to foster ethical, privacy-preserving AI in healthcare. By leveraging Federated Learning, we can empower hospitals and research institutions to collaborate without violating data privacy laws. This study is a step forward in enabling AI-driven medical research that respects both data security and scientific progress.
Looking Ahead As federated learning continues to evolve, FAIrPaCT will advocate for its adoption in medical AI, legal AI, and other privacy-sensitive domains. We encourage researchers and policymakers to explore Federated Random Forests as a viable solution to bridge data gaps while ensuring patient confidentiality.
Read the Full Paper: https://arxiv.org/abs/2405.20738
For more updates on ethical AI solutions, stay tuned to FAIrPaCT’s latest research and projects!