Federated Artificial Intelligence fRamework for PAncreatic Cancer Treatment
Federated Artificial Intelligence fRamework for PAncreatic Cancer Treatment
Undoubtedly we have no questions to ask which are unanswerable. We must trust the perfection of the creation so far, as to believe that whatever curiosity the order of things has awakened in our minds, the order of things can satisfy. Every man’s condition is a solution in hieroglyphic to those inquiries he would put.
Introduction
Pancreatic cancer is expected to be the second leading cause of cancer deaths in industrialized countries by 2030. Further, treatment is difficult because tumors are often resistant to conventional chemotherapy. The research network “FAIRrPaCT” is funded by the BMBF as part of the National Decade to Fight Cancer and wants to develop a software system that can individually predict the probability of success of pancreatic cancer treatments. The system the researchers want to develop is based on what is known as federated or distributed artificial intelligence. An algorithm that enables machine learning with data sources from different locations. “FAIRPaCT” uses three of the largest and most meaningful datasets of pancreatic cancer patients in Germany. These are the clinical data of the patient and the molecular data of the removed cancer cells. The data were obtained at the network clinics: at the University Medical Center Göttingen, at the University Hospitals of Giessen and Marburg, and at the Rechts der Isar Clinic of the Technical University of Munich.
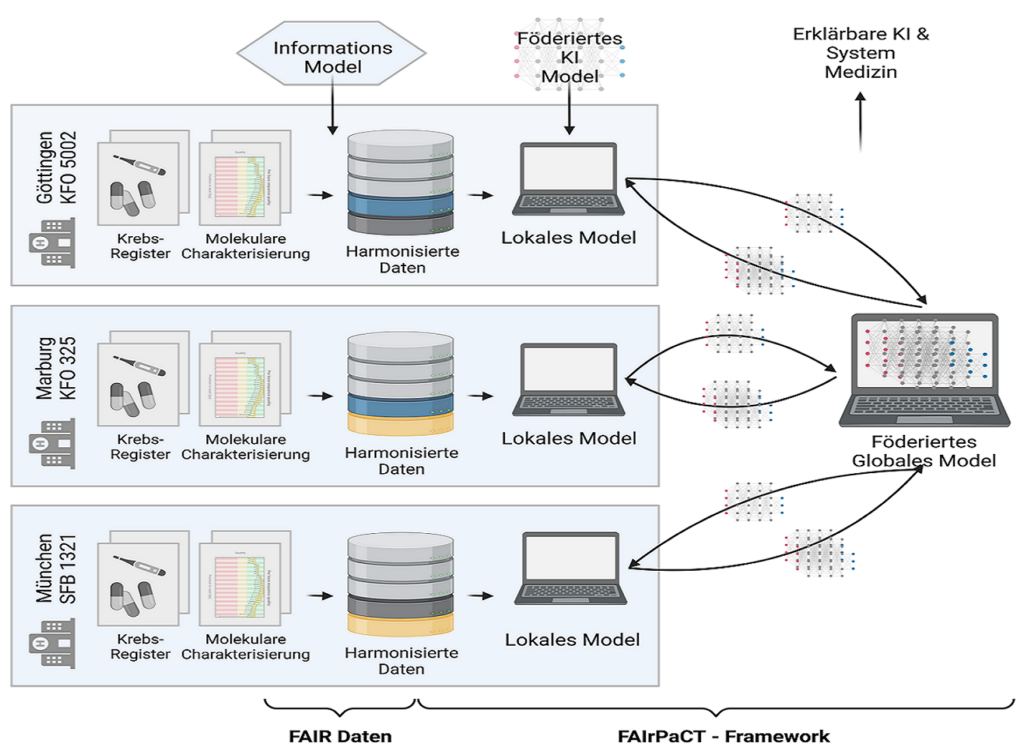
Goals
In order to develop an federated artificial intelligence (FAI) framework to predict and optimize the treatment of robust PDAC and address the project goals of the described oncological and computational challenges, the following objectives can be mentioned:
1. Development of a standard PDAC information model and data management for heterogeneous, non-independent and identically distributed (Non-IID), partially overlapping registry and omics data.
2. Identify, standardize/unify and disseminate the PDAC database based on FAIR criteria (Findable Accessible Interoperable Reusable).
3. Development of robust federated AI models for PDAC treatment prediction on Non-IID biomedical data.
4. Development of interpretable AI (xAI) methods for FAI ML models to identify relevant global clinical and molecular variables that drive treatment response.
5. Deploy distributed software system integrating DM, FAI and xAI PDAC frameworks
6. Dissemination of the information model, FAI algorithms, FAIrPaCT software framework, data and results to scientists, clinicians, patients and public.
Discussion
The software system that the research team wants to develop should be able to estimate the probability of success for specific treatment approaches that will be applicable regardless of location. Furthermore, it aims to identify key parameters that influence the response to a given treatment. This could be an important step towards AI-supported precision medicine.Data are obtained from molecular and biochemical analyses, modern imaging methods, clinical studies of diseases in patients. This treasure trove of data should be increased in the future. Therefore, new approaches to use data, advanced methods of artificial intelligence, machine learning and statistics to improve the analysis and extraction of research-related information are of great importance.
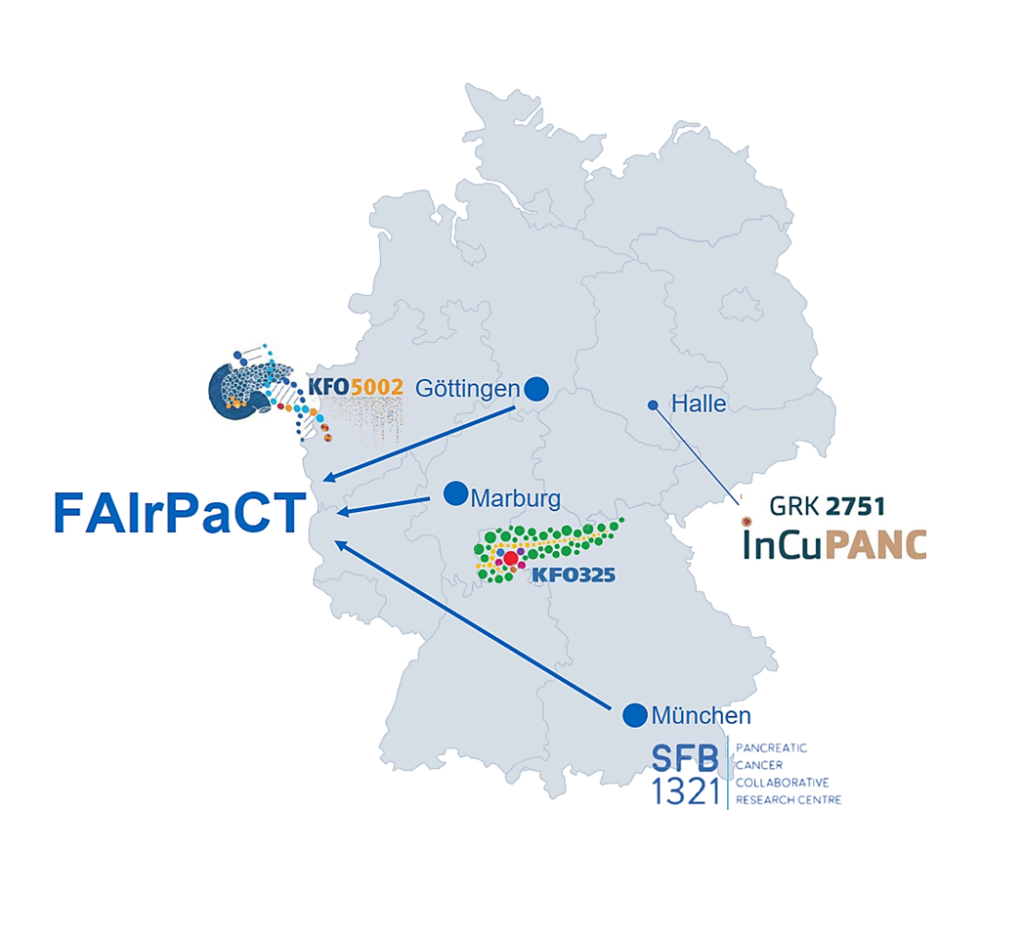
